The project is targeted to the public and focuses on improving the mental health and wellbeing of people within the environment of the display device. The project aims to fill in the existing gap for developing all-in-one digital therapeutic display systems by launching a digital platform with real-time communication and mobile phone sensors to promote health and wellbeing compatible with the latest healthy intelligent building ambitions, ethical AI, and digital health care technology protocols. The therapeutic digital display devices will provide content-based biophilic theory, personal circadian cycles, dynamic mood, and indoor environmental conditions. Within the first stage of the project, a biophilic classification function was developed to help artists classify their artwork into biophilic attributes in the TexturaTM, which is the device used to display the artwork.
Biophilic art, also known as nature-inspired art, is a type of artwork that incorporates elements of nature into its design. In recent years, deep neural networks have been used to classify biophilic art, allowing for more accurate and efficient categorization of this type of artwork. The process of using deep neural networks to classify biophilic art begins with the collection of a dataset of biophilic artwork. This dataset should include a diverse range of images, representing different styles and forms of biophilic art. Once the dataset has been collected, it is then split into two sets: a training set and a testing set.
The training set is used to train the deep neural network, which involves feeding the network a series of images and their corresponding labels (i.e., the category to which each image belongs). As the network is trained, it begins to learn the characteristics and features of biophilic art, allowing it to accurately classify new images. Once the network has been trained, it is then tested on the testing set to evaluate its performance. This involves feeding the network a series of images from the testing set and comparing the labels it assigns each image to the true labels. This allows us to determine the accuracy of the network and adjust its parameters as needed to improve its performance.
One of the key advantages of using deep neural networks for biophilic art classification is their ability to learn and adapt. Unlike traditional classification methods, which rely on pre-defined rules and categories, deep neural networks can learn and adapt to new data, allowing for more accurate and efficient classification of biophilic art.
In conclusion, deep neural networks offer a powerful tool for the classification of biophilic art. By leveraging the capabilities of these networks, we can more accurately and efficiently categorize this type of artwork, allowing us to better understand and appreciate the beauty of nature-inspired art.
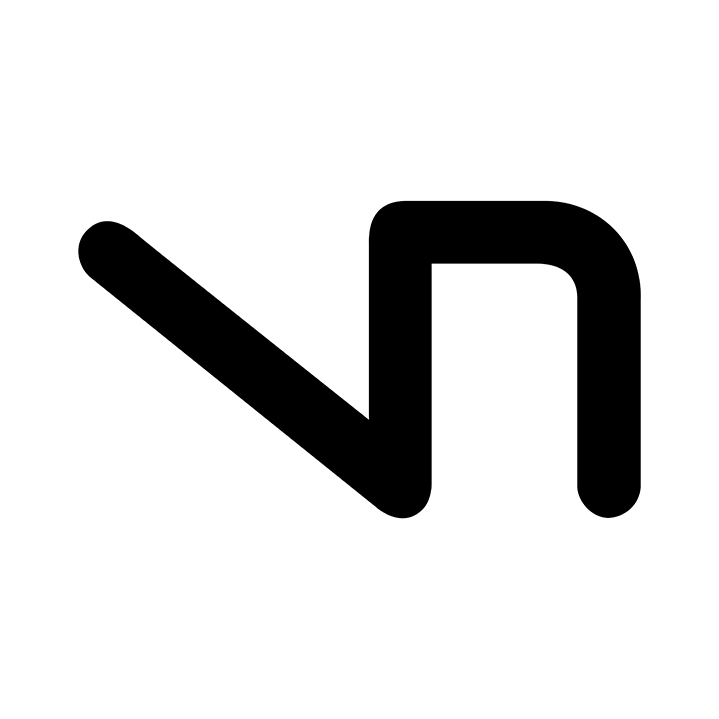
Anthony Adole is a KTP Associate for Vieunite. He specialises in Computer Science and Machine Learning.